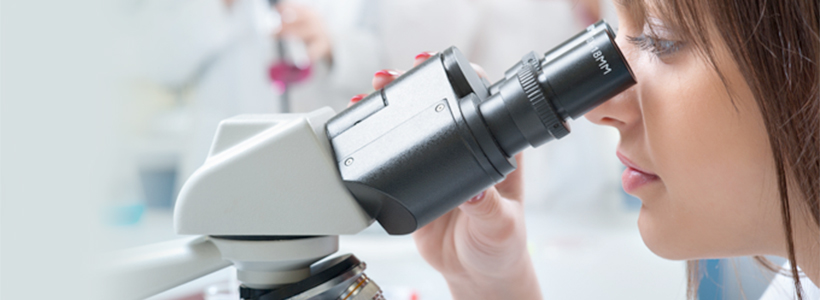
Climate change phenomena have been a global topic in environmental and water resources management. Nowadays weather is not as it used to be. High variability and persistence are common features of climatological time series data. Seasonality is one of the most key factors influencing variations in a time series. In several time series analysis, variations prompted by seasonal factor sometimes dictate the variations of the original series. This issue often occurs due to the environmental influence, such as periodic trend and variance clustering. Fourier based approach to seasonality modelling on vector autoregressive framework of rainfall and temperature series of Katsina was developed. An FtVAR(2) model for the series was able to capture the temporal linear properties of the two climate variables. However, it is shown that the bi-climate history is affected by nonlinear characteristics of the variance often referred to as variance clustering or volatility; this was captured by incorporating GARCH family model to the fitted FtVAR which resulted to FtVAR-mGARCH model. Comparing the efficiency of the two developed models, the FtVAR-mGARCH model performs better than FtVAR model arbitrating based onRMSE, MAE and MAPE of both models and therefore it’s best captured the dynamics of the climate variables. This result may give insight on the temporal dynamics of tropical continental weather variable for proper planning environmental and water resources management.